Data Sharing, Processing, and Management
Meteorology has a key role to play to improve energy sector activities at all timescales, from generation operation and dispatch, to risk management, to long-term resource assessment and to planning. This role is even more critical in the face of the energy transition, as with the increasing power generation from renewable sources, which have a higher sensitivity to climate variability and climate change. And in this context sharing data is key to develop, calibrate and validate useful energy models at regional or global scales. It has been demonstrated that when multiple data sources are used in models, the simulations and forecasts are better. This benefits the entire community including the energy user. However, processing and integrating different data sources is challenging as it implies managing large amount of data from Gigabytes (109 bytes) to Terabytes (1012 bytes) of information, which then needs to be checked, stored, analysed, and used by models and other (visual) applications, by tailoring it. Often, tailoring also implies simplifying the data and products so the user can receive a manageable amount of information, typically of the order of Megabytes (106 bytes) or Kilobytes (103 bytes) (Figure 1).
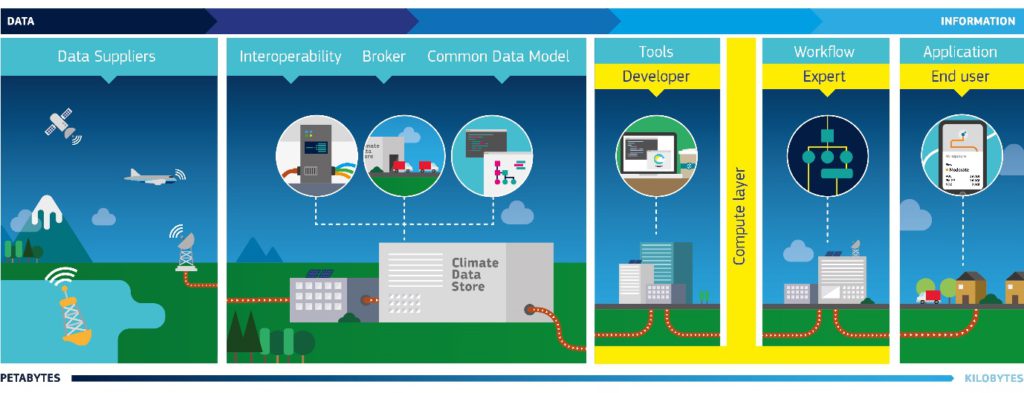
Figure 1. Data processing chain from real time measurements to the end user. Source: ECMWF
If increased interconnectivity and growth of hybrid grids is going to be the norm, there is a need to share information larger amount of data, for example those related to energy generation and demand under specific weather conditions. The benefits of doing so are many, for example aiding data simulations, training of Artificial Intelligence (AI) algorithms, helping to initialize real-time algorithms, and verifying models, essential for improving renewables forecasts, adequacy and climate risk assessments, resource identification and improve energy efficiency.
Common data issues
Although there are many initiatives aiming to make data available, there are still critical aspects that need addressing in terms of:
- Data availability
- Data quality
- Data readiness
To improve data availability, sharing data and observations coming from each component of the weather, climate and energy systems need to be considered:
- Meteorological and hydrological data: Satellite images, ground-based observations and historical data.
- Power generation data
- Energy demand data
- Energy usage data and its link with local conditions
Certified data quality assurance must also be a high priority as data that does not pass quality checks substantially increase inefficiencies in W&CS workflows, especially when in the end these data must be discarded. Also, it is not unusual that the quality of measurements degrade over time due to lack of maintenance of instrumentations, deteriorating conditions of the site, changes in software programmes used to handle the data, etc. (WMO SG-Ene, 2022).
Creating ready-to-use data following internationally agreed standards, to make them interoperable, traceable to its original source and quality controlled, is a time-consuming task that needs doing before any analysis can begin. Not addressing data issues in a timely manner can increase costs, increase workload, and degrade forecast quality (Haupt, 2019). This is especially important for databases which integrate information from different sources and with large amount of data, for example storing local observations as well as global reanalyses, the latter with sizes of the order of Terabytes, but up to Petabytes (i.e. 1015 byte).
Compiling data from a myriad of sources with quite different formats (e.g. point data, gridded or raster data, time series, nominal data, etc.) has its own challenges (WMO SG-Ene, 2022), some of which are:
- Different data formats
- Lack of metadata
- Low data standards
- Poor data accessibility
Data Management
To reduce repetitive and time-consuming tasks, it is important to properly handle data integration processes. To this end, Data Management Plans (DMPs) are essential to ensure operational systems can run efficiently and without (major) interruptions (Haupt 2019, WMO SG-Ene, 2022). They need to include:
- Basic project information (name, funding body, budget, duration, partners);
- Data management policies and guidelines, including access and data sharing;
- Clear standards for data, metadata and documentation (including standardised UTC time stamps): Inconsistent timestamps, not clarifying if UTC has been used versus a local time, failing to clarify if the time stamp considers changes for daylight savings or not. Some utilities span multiple time zones, and in many cases, the time zone on the data depends on which time zone data was downloaded, rather than the location of the data source.
- Clear methods for pre-processing and data cleaning
- Standardized data formats allowing for interoperability between data sets: Interoperable systems and devices can exchange data and interpret said shared data. They also must be able to present that data in a way that can be understood by users regardless of their physical architecture and operating systems.
- Dedicated data storage for easy data sharing and access
- Reliable storage, security, backups and long-term preservation of data
- Solutions for an efficient pre-processing as part of data queries in mega databases to reduce the size of data download
- Clear economic costs and ways of financing short-term and long-term storage
- Regular DMP revisions assigning responsibility and due dates.
Data sharing
Global cooperation in data sharing is key as there is a correlation between the width of the window into the future of the forecast and the spatial coverage of the training data (regional vs global). So, for seasonal forecasts, and perhaps even more so for climate projections, accurate and detailed time series coming from an evenly distributed network of sensors around the globe would be ideal. Moreover, data exchange programmes should be developed based on conventions compliant with the FAIR principles: FAIR Guiding Principles for scientific data management and stewardship were published in Scientific Data. The authors intended to provide guidelines to improve the Findability, Accessibility, Interoperability, and Reuse of digital assets.
Also, the contribution to data sharing from private companies is really important. Often these data are considered as financially or operationally sensitive. In such cases, to protect private companies interests, non-disclosure agreements could be signed with the entities receiving their data, so as to allow using their data to train algorithms and models, but without being directly accessed by other users.
WMO participation in data-sharing initiatives
started around 1960, and has 193 members consisting of national weather services, marine services, space agencies etc. It implements the Global Observing System (GOS). WMO functions as the regulatory body to ensure standardization with elements such as what is being measured, where, how often and how that information is being exchanged internationally.
which has mandatory special resolution requirements and a mandatory reporting frequency. However a stronger commitment from all parties is needed as only few complying with the agreement in terms of the frequency of reporting. To fill in areas where there is a gap on data reporting an US$350m capital investment is needed with US$150m in annual operating cost that could be funded by entities such as the World Bank.
References
- Dubus, L., Muralidharan, S., Troccoli, A. (2018). What Does the Energy Industry Require from Meteorology?. In: Troccoli, A. (eds) Weather & Climate Services for the Energy Industry. Palgrave Macmillan, Cham. https://doi.org/10.1007/978-3-319-68418-5_4
- Haupt, S.E. 2019 Data Exchange, Access and Standards Special Interest Group (Data SIG) [Webinar] WEMC Data SIG public Webinar 13/06/2019
- WMO. 2015. Policy for the International Exchange of Climate Data and Products to Support the Implementation of the Global Framework for Climate Services. Resolution 60 (Cg-17).
- WMO SG-Ene. 2022. Weather & climate information production In: Guidelines of the WMO Commission for Weather, Climate, Water and Related Environmental Services and Applications- Study Group on Integrated Energy Services, Integrated weather & climate services in support to net zero energy transition.
- Wilkinson, M., Dumontier, M., Aalbersberg, I. et al. 2016. The FAIR Guiding Principles for scientific data management and stewardship. Sci Data 3, 160018.